How We Proved a Major Insurer Could Leverage Geospatial Data to More Effectively Mitigate Property Risk
When a company makes its money by managing or mitigating risk, any improvements to efficiency that enhance already small margins can make a big difference.
It’s why a leading insurance group wanted to bolster its property risk assessment and underwriting processes by more effectively identifying specific property attributes and potential liabilities. One way to do this would be to automatically acquire and analyze geospatial data of geographies and individual properties, powered by machine learning (ML).
If successful, this could deliver transformative benefits across the insurance policy lifecycle, from policy issuance through to claims management. It would control costs by reducing physical visits by risk assessors and claims adjusters, to enable more competitive premiums and accelerate claims payouts to enhance customer satisfaction.
This insurer had already identified a significant opportunity to generate its chosen property attributes in-house using Google Earth Engine and Google Cloud, rather than relying on individual data vendors. It then approached SoftServe, due to our status as a Premier Consulting partner to Google Cloud, to suggest how to make this work most effectively.
Specialist expertise
Until then, and because the insurer operated across multiple geographic locations, it was relying on a fragmented approach to obtain geospatial data from various sources. It had realized that the existing approach would benefit from automation to improve efficiency and accuracy.
Experience showed that a fragmented approach across different countries was time-consuming and prevented full utilization of automation capabilities. However, the insurer was told that a solution could be developed by SoftServe that had proven expertise working with structured and unstructured data, including geospatial. So, it decided to partner with SoftServe and Google Cloud to build a solution use case and validate the results.
Single source of data
To move forward and improve risk management and underwriting by utilizing accurate geolocation information, it therefore first needed to create a single source of geospatial data. This meant being able to process large schedules of locations, boosting the processing of pricing, and reducing reliability on third parties.
The main goal was to successfully deliver feasible, high-quality outputs from geospatial data for 12 selected attributes across a subset of a sample property portfolio. This would initially cover at least 25,000 locations, comprising over 7,000 sites in each of the three geographic areas provided.
SoftServe’s initial goal was to successfully deliver in six weeks a proof of concept (PoC) focused on generating outputs for the 12 attributes across the portfolio subset. The attributes the client required to be included in the PoC were:
- Distance to closest building outside parcel
- Distance to closest building inside parcel
- Height of building
- Solar panels presence
- Solar panels area
- Occupancy type
- Vegetation density
- Area of the building
- Area of the parcel
- Roof tree overlap
- Ground slope
- Roof surface area
By incorporating this level of micro-detail from the geospatial evidence, the insurer would be able to more accurately improve its pre-insurance risk assessments by analyzing those attributes before issuing policies. It would enable the risk department to predict risk at the point of sale, ensuring they have a much clearer understanding of their exposure before insuring a property.
Previously, if you wanted to insure your house by just providing an address, the insurer would assess the potential risk based on known local concerns. However, by adding analysis of the key geospatial attributes, it can make more informed underwriting decisions more quickly.
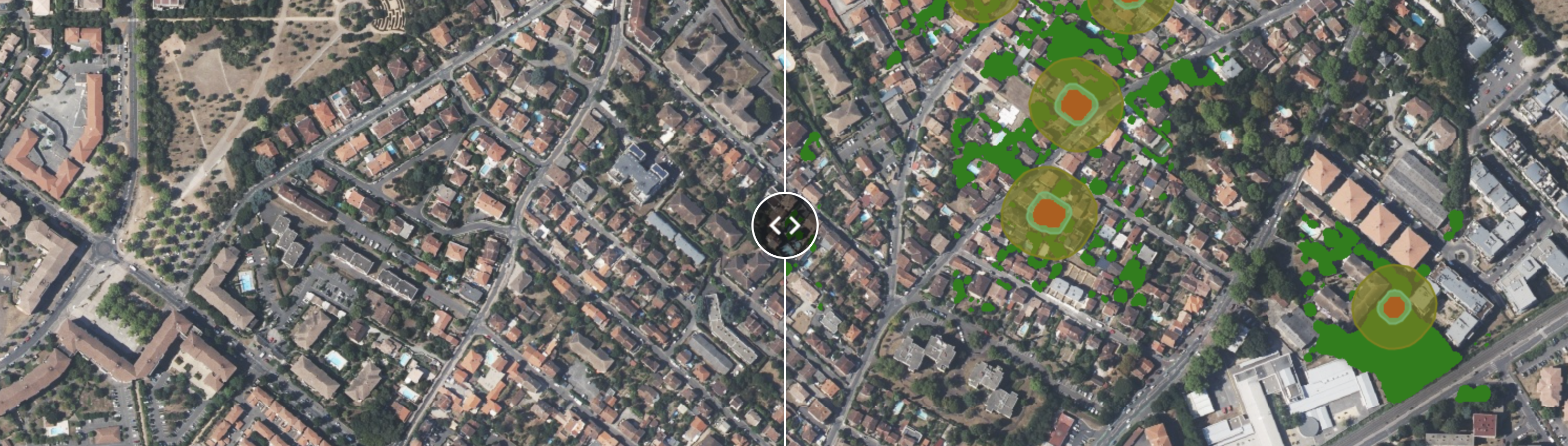
Building the proof
To tackle this project, SoftServe deployed a small specialist team that started by reviewing publicly available datasets to identify those most applicable for automated attribute detection. If successful, SoftServe then proposed scaling this PoC across the client’s global property portfolio, including further attributes and additional countries.
The datasets were used as the foundation for computer vision models and analytical functions that determine property characteristics based on selected attributes. The main project activities included:
- Research to create a solution scalability vision document.
- Data collection and preprocessing model development
- Tuning and attributes extraction
- ML segmentation model training for solar panels and vegetation
- Integration and processing of geospatial datasets for non-ML-based attributes
- Running ML models on input locations provided by the client
- Validation and presentation
Despite some challenges with initial source data, SoftServe successfully achieved the primary objective of demonstrating the feasibility of the Google Cloud capabilities and SoftServe’s models. This meant it was able to deliver the three main conclusions:
- Generate outputs in the format requested for priority attributes.
- Provide a scalable strategy for extending the PoC.
- Estimate cloud operating costs from a fully deployed solution.
PoC goals achieved
The PoC proved that the geospatial improvements would lead to process optimization by ensuring an enhancement of portfolio management with accurate geolocation information. This would be underpinned by efficient calculations for risk analysis due to a complex approach to get spike insights of joined metrics.
It also showed that if the client implemented the computer vision model and a unified automation approach, it would significantly enhance underwriting with more accurate property risk pricing.
Our PoC reached the main project goal to show the feasibility of geospatial analysis through Google Cloud technologies and SoftServe’s models. It also showed that further implementation would allow the approach to be scaled and underwriting improved. Most importantly, it demonstrated that implementing an in-house geospatial solution would enable the client insurer to optimize costs and achieve more accurate pricing.