How Machine Learning Drives Artificial Lift Performance
DURATION:
51 min
FORMAT:
AWS,Presentation
Unplanned downtime in the oil and gas industry leads to costly problems like production delays. With artificial lift downtime being a primary source of such deferred production, identifying potential problems before they occur is crucial.
By applying advanced analytics and machine learning (ML) at scale in the cloud, oil and gas companies can improve their field performance. Using asset diagnostics and acting on real-time monitoring insights, you’ll create data-driven maintenance flows, leading to an increase in production and reduced lease operating expenses.
This video explains how AWS production monitoring solutions and services like Amazon SageMaker reduce unscheduled maintenance and deferred production. These tools help you to predict suboptimal equipment performance and potential failures so you can make data-driven operational decisions.
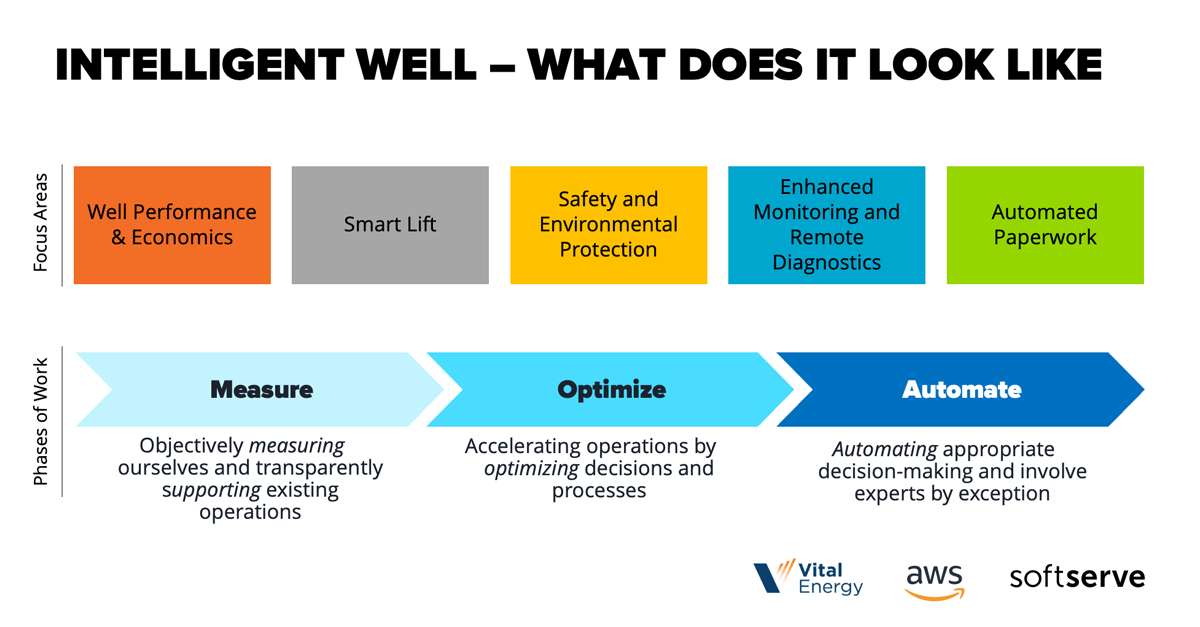
Jetzt Video ansehen
Weitere Videos
Engage with SoftServe’s industry and technology experts at meetups, conferences and online events
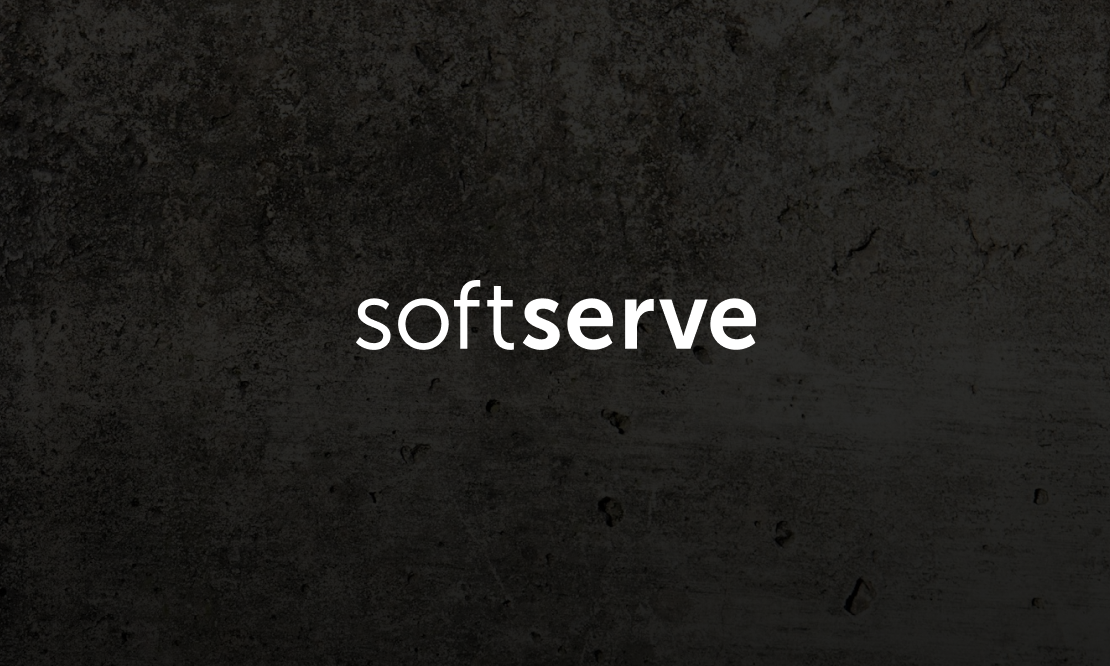
AI’s Role in the Future of Oil & Gas
Energy Sector,Energy, Oil & Gas,Tech Talks
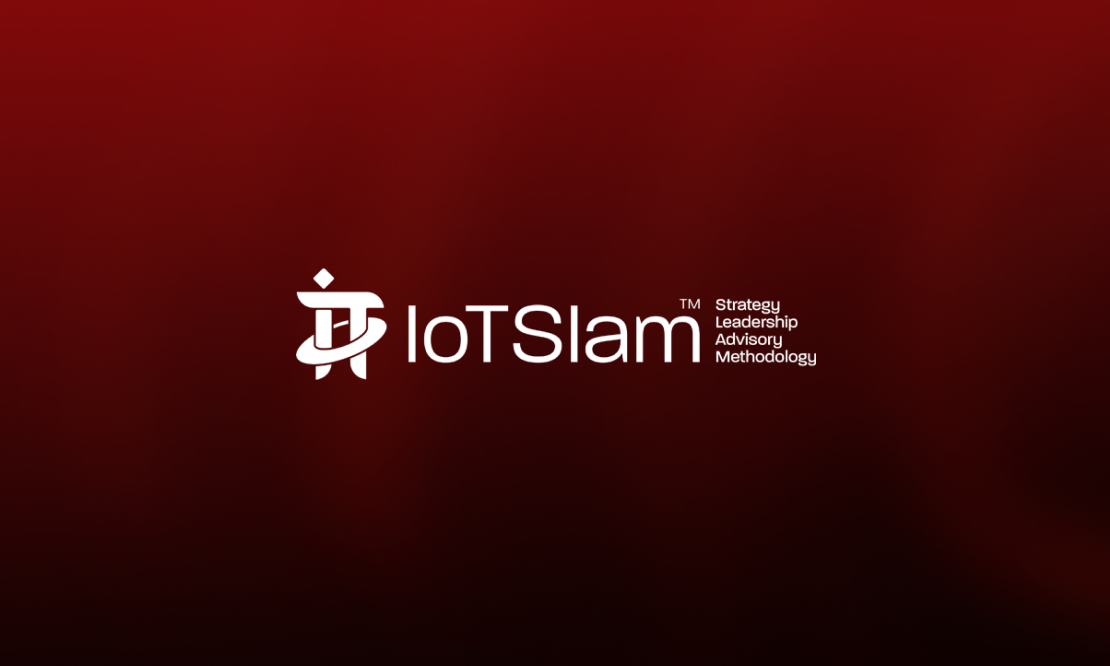
Look Under the Hood: Edge Solutions in Practice
Manufacturing,Panel Discussion,Supply Chain
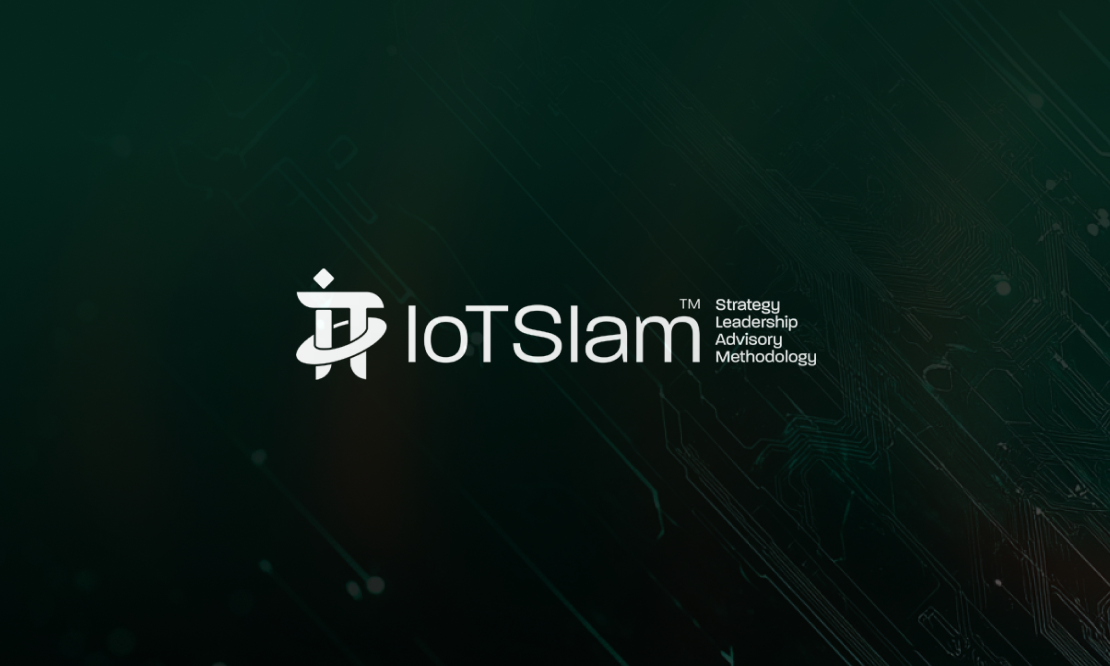
Navigating Edge Technology in Manufacturing
Manufacturing,Panel Discussion,Supply Chain
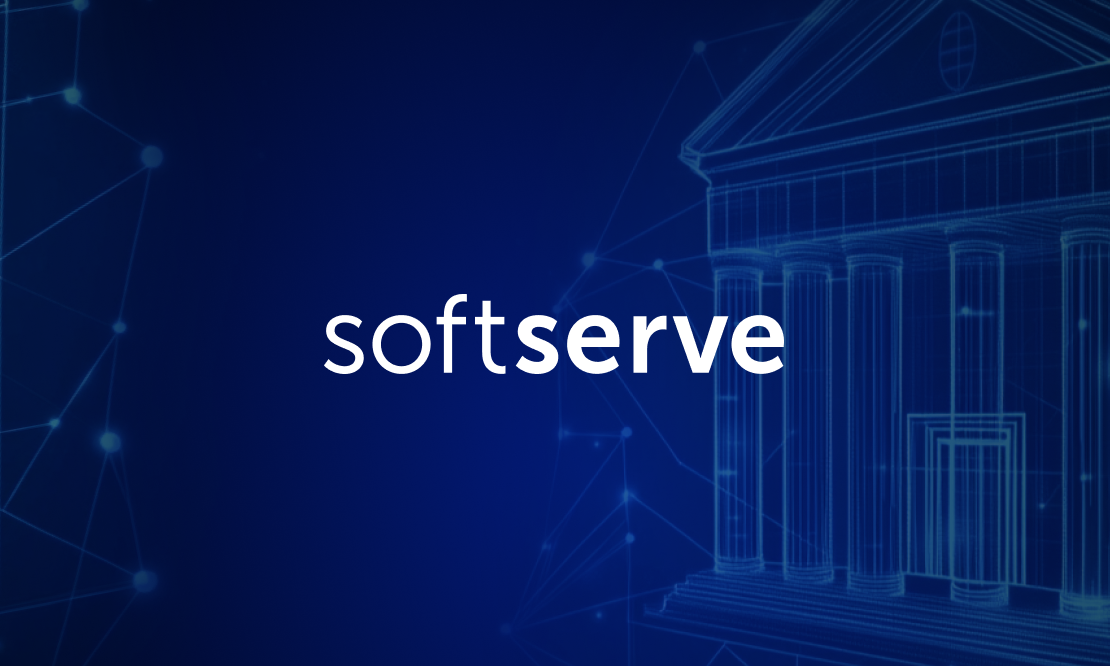
Beyond Automation: Unleashing the Power of IoT and Generative AI ...
Interview,Manufacturing