Interactions between proteins are a fundamental part of many biological processes, with a significant part of them involving peptides. A chemical similarity with proteins, had recently ignited much attention to peptides as promising drug candidates. Therefore, the design of peptide-derived therapeutics heavily relies on underlying protein-peptide interactions. Molecular docking is one of the most frequently used methods, which predicts a preferred pose of one molecule (known as a ligand) with respect to another (receptor), when they form a stable complex. Methodologically, docking often consists of two stages: proposal of a possible receptor-ligand pose; and it’s evaluation by a scoring function.
SoftServe proposes an end-to-end molecular docking pipeline, as shown schematically in Figure 1 below. The first stage involves a deep learning model, predicting the ligand’s docking spot on the receptor. A neural network takes into account atomic sorts, intramolecular bonds of both receptor and ligand, and predicts a region near receptor, where a ligand will attach itself upon docking.
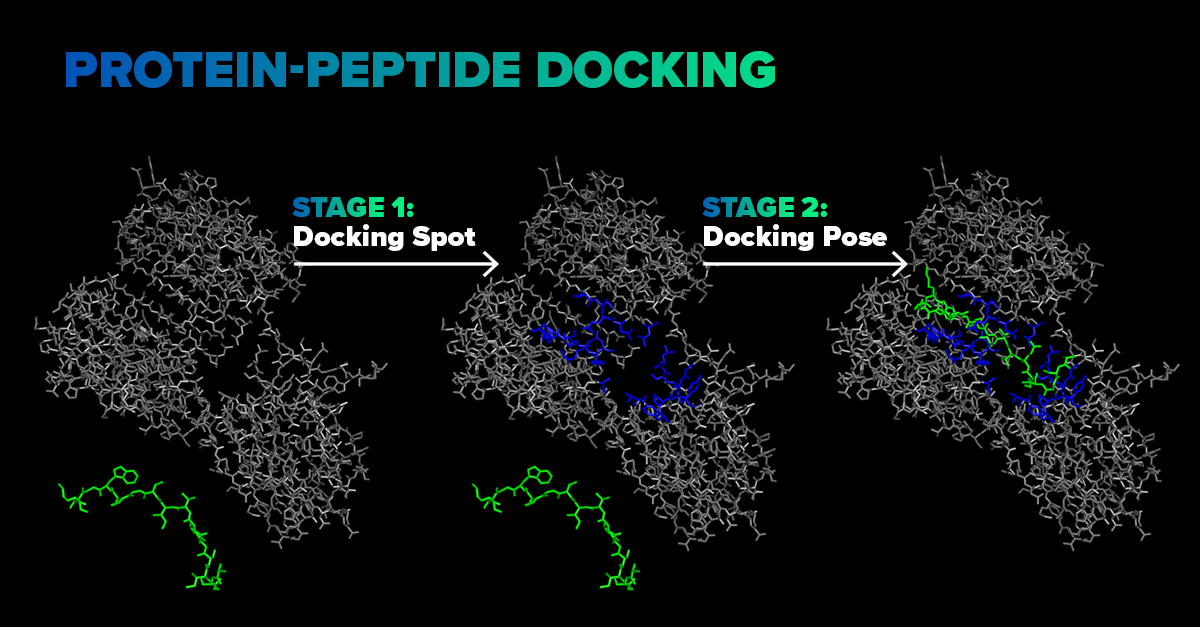
Figure 1: A schematic representation of the docking pipeline. First stage – quick localization of a docking spot, second stage – quantum mechanical calculation within the docking spot to fine-tune the docking pose.
Such a prediction takes seconds and serves as a quick estimation to shrink the search space. We designed this model from scratch and trained it on protein-peptide complexes from the PepBDB dataset. The second stage is not fully automated yet; however, at this stage the ligand’s position and conformation is fine-tuned. For this purpose, the ligand is automatically placed in front of the predicted docking spot and DFTB+ — a Density Functional Theory (DFT) based quantum mechanics simulation is performed for the final ligand conformation discovery, as well as the binding energy calculation.
The developed end-to-end pipeline combines deep learning and DFT approaches to predict protein-peptide docking. It must be noted that peptides is not the only a class of possible ligands since our solution can also dock other organic compounds. A strong advantage of the approach is the provision of a two-stage set-up, allowing one to localize the docking spot as a first stage, thus, sufficiently reducing the calculation time and expenses; while, the high precision rate is kept with the power of DFT during the second stage.