The Goldilocks Principle: Retail Inventory Optimization Through Predictive Demand Forecasting
How to use machine learning to set your stock levels just right — avoiding stockouts, reducing excess inventory, and building retail agility
As the age of supply chain disruption-as-a-rule continues unabated, retailers can't afford to have their operating capital tied up in inventory. Unfortunately, many still find themselves saddled with excessive stock unaligned with current demand. The reason? Faced with a lack of robust retail demand forecasting and fearing revenue losses due to stockouts, many retailers end up with inventory mismatches.
To avoid resource misallocation, it’s crucial to get inventory levels just right — high enough to satisfy consumer demand, but not so high that unsold items occupy shelf space in stores or accrue storage fees in warehouses. Increasingly complex consumption dynamics spread over multiple channels make determining optimal stock levels a tall order. But what if you could boost the accuracy of your demand forecasting by up to 20% — enabling precise stock management and reducing inventory costs?
That’s now eminently achievable, thanks to advances in machine learning (ML). Built using the data you already collect and enriched with external sources, ML algorithms deliver the robust demand predictions you need to safely avoid overstocking, free up working capital, and grow your retail operation’s resilience.
Overstocking: The lesser of two evils?
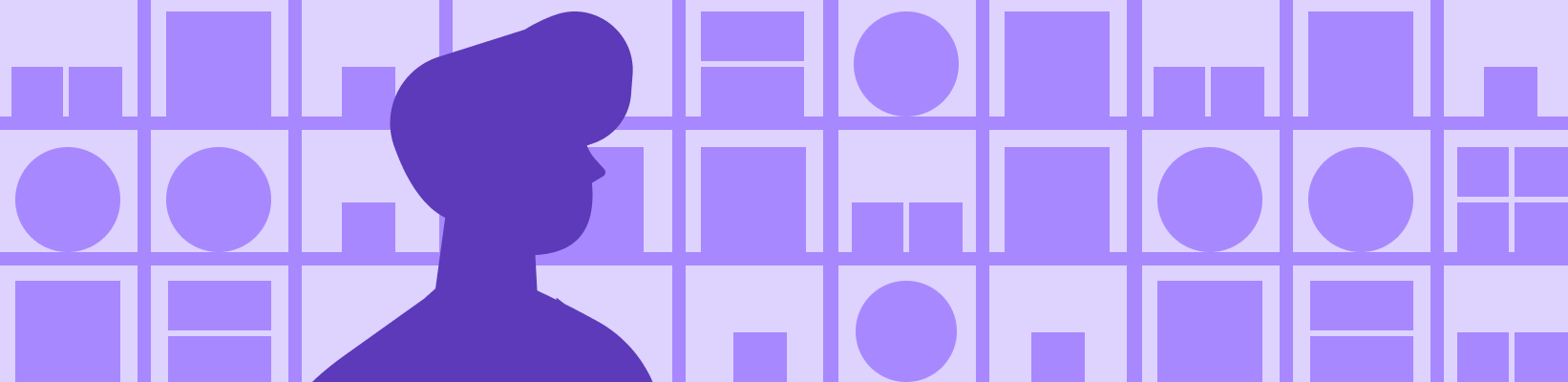
Flexibility is paramount to enterprise resilience, and pivoting requires the ability to redirect funds. Yet many retailers still find themselves burdened by overstocked warehouses, severely restricting their working capital and weighing them down with pricey storage fees. The reason is that, in the tight confines of retail stores, space is a premium, which leads to a dependency on warehouses for surplus stock — a clear sign of gaps in retail stores inventory optimization. Without the ability to precisely predict the what, where, and when of consumer demand, retail stores can struggle to maintain ideal stock levels.
Missed revenue and excessive carrying costs: A product of outdated demand forecasting
So, how can retail companies reduce inventory and free up their capital while also guaranteeing in-stock products and satisfying consumer demand?
Consider the example of an American hardware retailer. Operating across several regions, the company had to regularly re-stock its stores from distribution centers. Their replenishment strategy relied on store managers’ planning and retail demand forecasting methods using static statistical models to determine the necessary inventory levels.
Optimized inventory with predictive demand forecasting
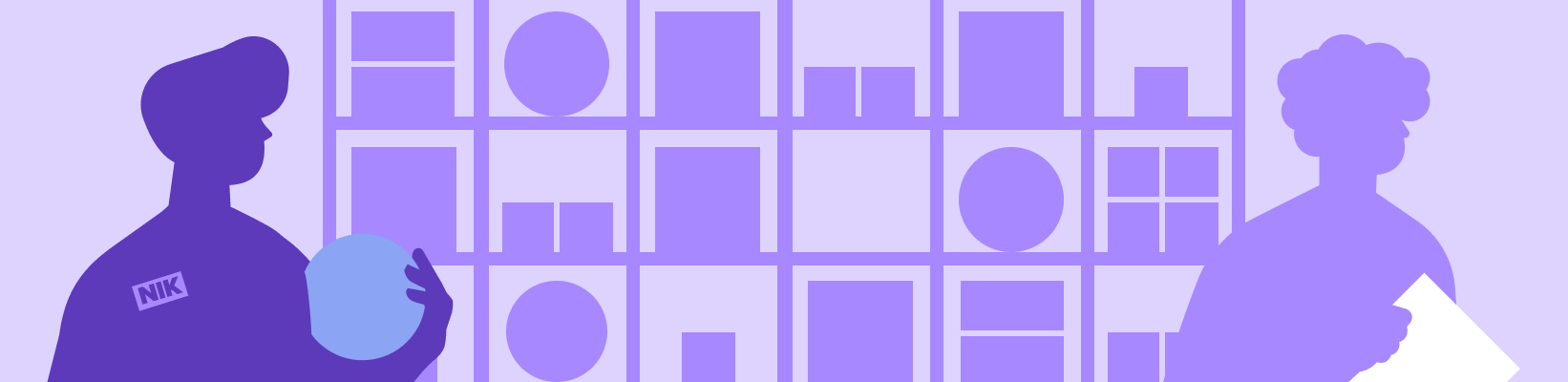
In the face of overstocked products locking up cash and floor space, the hardware retailer partnered with SoftServe to build a better method of forecasting demand for their most important product categories. The result was a predictive, ML-driven demand forecasting algorithm.
Based on a data pipeline including the company’s internal data from historical sales, inventory, and purchase orders, the algorithm was enhanced by external factors such as local events, alongside analytics accounting for periodic and seasonal variations.
In a further step, the algorithm proposes a reorder level and a minimum reorder quantity based on batch size and expected delivery. Using its more advanced demand forecasting, the hardware company is poised to free up over $10 million annually thanks to:
- 12.5% reduction of inventory levels without sacrificing revenue
- 20% greater forecast accuracy compared with statistical models
- Three-month maximum forecasting horizon for reliable predictions
ML-driven demand forecasting: The key to an increasingly complex retail environment
Conventional forecasting methods were created for a simpler time. In an uncertain environment dominated by supply chain instability and increasingly complex customer demands and buying patterns, they are not up to the task. Armed with ML-driven predictive demand forecasting drawing from internal and external data sources, retailers are positioned to optimize stock management, free up working capital, and secure profits by satisfying consumer demand.
With a wealth of experience in deploying AI/ML solutions for retail inventories across all major cloud providers or on-premises, SoftServe is the technology partner that can help you quickly deploy a robust, intelligent, data-driven demand forecasting solution for your business. That builds resilience and agility into your operations.
Don’t hesitate to learn more about SoftServe’s offering from our demand forecasting demo page.
Or, if you are interested in starting your own intelligent demand forecasting solution — Let’s talk!
Start a conversation with us