Bring AI to Your Data and Have Your Data Talk to You
It’s safe to say that nearly everyone is interested in AI and many of them are looking closely at Generative AI.
It’s also fair to say that plenty of them are even starting to invest in these new technologies. But that doesn’t mean they are completely convinced it will deliver business benefits.
Many businesses have concerns about its safety, while others worry about the costs, or that they don’t have the skills to introduce AI effectively.
So that’s why we brought together a group of executives from the financial services industry for a workshop to talk through all the issues. Experts from Dell Technologies, NVIDIA, and our own team at SoftServe began by presenting how and where AI could make a difference and then led an open discussion to deal with specific questions in more detail.
What is possible?
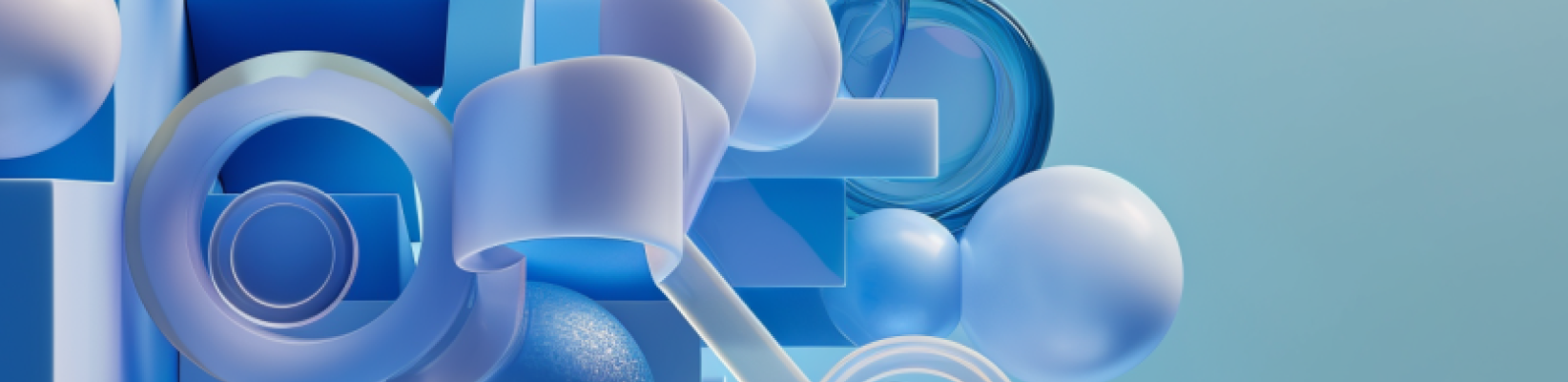
Most of all, people wanted to know what their peers were doing, where were the specific use cases, what should their priorities be, where should they start, what infrastructure is needed, and could we cut through the hype and tell them what is possible? They asked:
- How do we level up AI and Gen AI skills?
- How do we minimise risks?
- How do we maintain operational efficiency?
- How do we do this in a responsible manner?
- How do we get started?
Not a small ask when you only have a couple of hours. But they gave it a good shot.
The first thing to acknowledge is that AI is not new and has been around in various forms for decades. What has changed recently has been the ability to deploy AI economically and at scale. It can accelerate data processing capabilities that transform data analysis into actionable insights.
At the workshop, the overriding common denominator was the importance of data quality as the key to a successful AI strategy. “No data, no AI” was the simple mantra. So, starting with clean data is a must. A survey from CIO magazine was cited showing that 80% of AI initiative failures are down to the combination of a lack of governance, ballooning costs, unrealistic expectations, and overly complex data issues.
Put simply, these projects did not fail because of the technology, but due to a failure of expectations for what the AI was supposed to deliver.
We were told it was therefore critical to begin by defining the expected value outcomes and to fully understand the business initiative priorities. To start with these, we should focus on three main areas where AI has already been proven to succeed in financial services: customer experience; compliance and operational risk; and credit risk.
The next step should be to understand available resources and acknowledge that it is difficult to find people with the right skills. It means starting with engineers and building up to data scientists, as the latter needs serious supervision and guidance. “AI only succeeds when people, processes, and technology are in synch and harmony.” That means beginning with a policy of buying tech and maturing to a level where building it becomes possible. It also means that you should bring AI to your data, not the other way around.
Feasibility
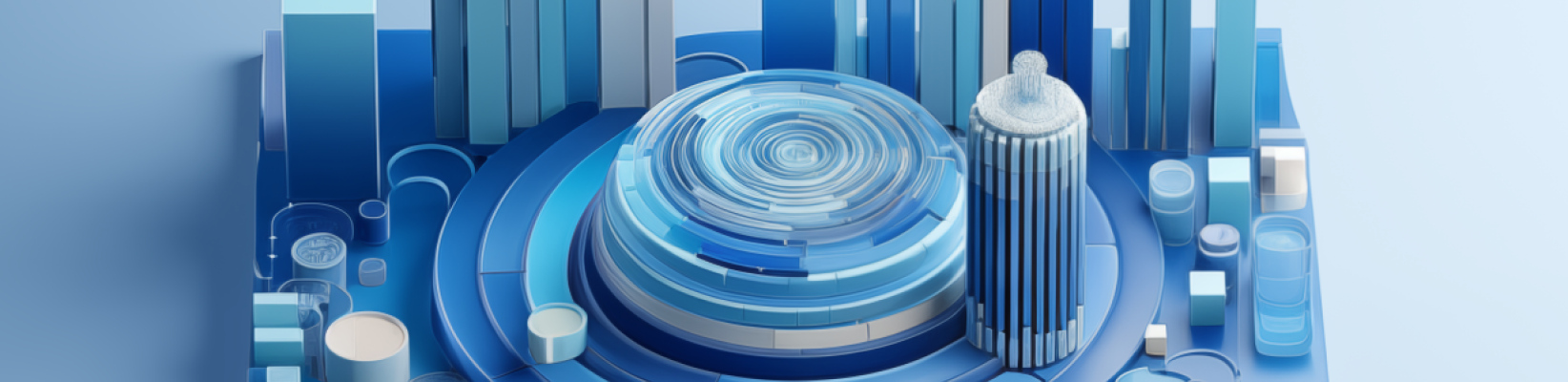
So, once a strategy and focus have been established, business value and feasibility need to be assessed, both in terms of actual cost and ROI. “AI is not cheap, nor are the GPUs that drive it.” For example, it was important to understand the size of GPU you will need to use to run the model required to deliver the desired outcomes. Here there was encouragement for some, as it was noted that today some large language models can be much cheaper to run on-premises.
Delivering reality
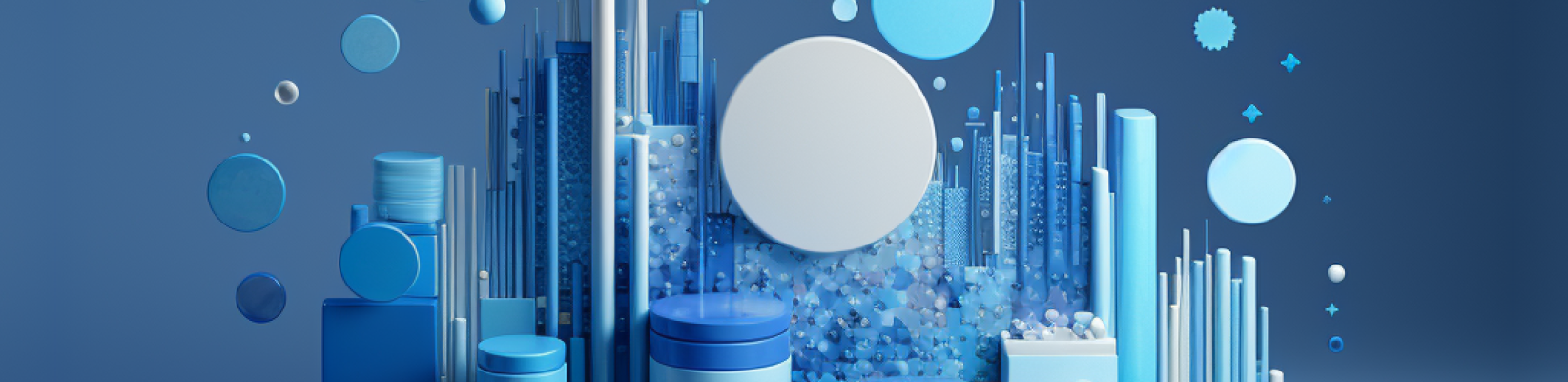
The final piece of the jigsaw was translating an AI project into reality. Here both Dell and NVIDIA emphasised the importance, particularly given the acknowledged AI skills gaps, of working with a firm like SoftServe to ensure implementation expertise.
You can have all the right hardware, software, models, and microprocessors, but if they are unable to talk to each other and make sense of the data, the outputs will be worthless. History has taught us that even great innovations in construction and industry have often taken many years (or decades) to deliver optimum benefits as new concepts were often not matched by practical implementation.
That ability to see what the business is trying to achieve, understanding what the technology can deliver, and providing the glue that binds the two together is key to a successful outcome. Experts who understand both the AI technologies and the practical business challenges of specific industry verticals like finance can be the difference between success and failure.
Therefore, if you want to talk to your data and have it talk to you — you should talk to us first.